- 快召唤伙伴们来围观吧
- 微博 QQ QQ空间 贴吧
- 文档嵌入链接
- <iframe src="https://www.slidestalk.com/u227/Guessing_a_real_valued_random_variable?embed" frame border="0" width="640" height="360" scrolling="no" allowfullscreen="true">复制
- 微信扫一扫分享
Guessing a real-valued random variable
展开查看详情
1 . Predicting Quantitative Features: Regression 36-350, Data Mining 6 October 2008 Reading: sections 6.1–6.3 and 11.1 in Principles of Data Min- ing. Optional Reading: chapter 1 of Berk. We’ve already looked at some examples of predictive modeling in the form of classification and factor analysis, but now we’ll get into it more seriously, and it will largely occupy us for the rest of the course. The place we’ll begin is with predictive quantitative features, i.e., regression. The math here is not so bad, and it connects more smoothly with your previous statistics courses; having learned some lessons here we’ll come back to classification, and then go to more complicated kinds of prediction. 1 Guessing the Value of a Random Variable We have a quantitative, numerical feature, which we’ll imaginatively call Y . We’ll suppose that it’s a random variable, and try to predict it by guessing a single value for it. (Other kinds of predictions are possible — we might guess whether Y will fall within certain limits, or the probability that it does so, or even the whole probability distribution of Y . But some lessons we’ll learn here will apply to these other kinds of predictions as well.) What is the best value to guess? Or, more formally, what is the optimal point forecast for Y ? To answer this question, we need to pick a function to be optimized, which should measure how good the guesses are — or equivalent how bad they are, how big an error is involved. A reasonable start point is the mean squared error: 2 MSE(a) ≡ E (Y − a) (1) So we’d like to find the value r where MSE(a) is smallest. 2 MSE(a) = E (Y − a) (2) 2 = (E [Y − a]) + Var [Y − a] (3) 2 = (E [Y − a]) + Var [Y ] (4) 2 = (E [Y ] − a) + Var [Y ] (5) 1
2 . dMSE = 2 (E [Y ] − a) + 0 (6) da 2(E [Y ] − r) = 0 (7) r = E [Y ] (8) So, if we gauge the quality of our prediction by mean-squared error, the best prediction to make is the expected value. 1.1 Estimating the Expected Value Of course, to make the prediction E [Y ] we would have to know the expected value of Y . Typically, we do not. However, if we have sampled values, y1 , y2 , . . . yn , we can estimate the expectation from the sample mean: n 1 r≡ yi (9) n i=1 If the samples are IID, then the law of large numbers tells us that r → E [Y ] = r (10) and the central limit theorem tells us something about how fast the convergence is (namely the squared error will typically be about Var [Y ] /n). Of course the assumption that the yi come from IID samples is a strong one, but we can assert pretty much the same thing if they’re just uncorrelated with a common expected value. Even if they are correlated, but the correlations decay fast enough, all that changes is the rate of convergence. So “sit, wait, and average” is a pretty reliable way of estimating the expectation value. 2 The Regression Function Of course, it’s not very useful to predict just one number for a feature. Typically, we have lots of features in our data, and we believe that there is some relation- ship between them. For example, suppose that we have data on two features, X and Y , which might look like Figure 1. The feature Y is what we are trying to predict, a.k.a. the dependent variable or output or response, and X is the predictor or independent variable or covariate or input. Y might be something like the profitability of a customer and X their credit rating, or, if you want a less mercenary example, Y could be some measure of improvement in blood cholesterol and X the dose taken of a drug. Typically we won’t have just one input feature X but rather many of them, but that gets harder to draw and doesn’t change the points of principle. Figure 2 shows the same data as Figure 1, only with the sample mean added on. This clearly tells us something about the data, but also it seems like we should be able to do better — to reduce the average error — by using X, rather than by ignoring it. 2
3 . 1.0 0.8 0.6 y 0.4 0.2 0.0 0.0 0.2 0.4 0.6 0.8 1.0 x plot(all.x,all.y,xlab="x",ylab="y") axis(1,at=all.x,labels=FALSE,col="grey") axis(2,at=all.y,labels=FALSE,col="grey") Figure 1: Scatterplot of the example data. (These are made up.) The axis commands add horizontal and vertical ticks to the axes to mark the location of the data (in grey so they’re less strong than the main tick-marks). This isn’t necessary but is often helpful. The data are in the example.dat file. 3
4 . 1.0 0.8 0.6 y 0.4 0.2 0.0 0.0 0.2 0.4 0.6 0.8 1.0 x Figure 2: Data from Figure 1, with a horizontal line showing the sample mean of Y . 4
5 . Let’s say that the we want our prediction to be a function of X, namely f (X). What should that function be, if we still use mean squared error? We can work this out by using the law of total expectation, i.e., the fact that E [U ] = E [E [U |V ]] for any random variables U and V . 2 MSE(f (X)) = E (Y − f (X)) (11) = E E (Y − f (X))2 |X (12) 2 = E Var [Y |X] + (E [Y − f (X)|X]) (13) When we want to minimize this, the first term inside the expectation doesn’t depend on our prediction, and the second term looks just like our previous optimization only with all expectations conditional on X, so for our optimal function r(x) we get r(x) = E [Y |X = x] (14) In other words, the (mean-squared) optimal conditional prediction is just the conditional expected value. The function r(x) is called the regression func- tion. This is what we would like to know when we want to predict Y . 2.1 Some Disclaimers It’s important to be clear on what is and is not being assumed here. Talking about X as the “independent variable” and Y as the “dependent” one suggests a causal model, which we might write Y ← r(X) + (15) where the direction of the arrow, ←, indicates the flow from causes to effects, and is some noise variable. If the gods of inference are very, very kind, then would have a fixed distribution, independent of X, and we could without loss of generality take it to have mean zero. (“Without loss of generality” because if it has a non-zero mean, we can incorporate that into r(X) as an additive constant.) This is the kind of thing we saw with the factor model. However, no such assumption is required to get Eq. 14. It works when predicting effects from causes, or the other way around when predicting (or “retrodicting”) causes from effects, or indeed when there is no causal relationship whatsoever between X and Y . It is always true that Y |X = r(X) + η(X) (16) where η(X) is a noise variable with mean zero, but as the notation indicates the distribution of the noise generally depends on X. It’s also important to be clear that when we find the regression function is a constant, r(x) = r0 for all x, that this does not mean that X and Y are independent. If they are independent, then the regression function is a constant, but turning this around is the logical fallacy of “affirming the consequent”.1 1 As in combining the fact that all human beings are featherless bipeds, and the observation 5
6 .3 Estimating the Regression Function We want to find the regression function r(x) = E [Y |X = x]. suppose that we have a big set of pairs (x1 , y1 ), (x2 , y2 ), . . . (xn , yn ). Then this is a supervised learning problem — we know the label (value of Y ) for each of our n train- ing points. How can we estimate the regression function from these training examples? If X takes on only a finite set of values, then a simple strategy is to use the conditional sample means: 1 r(x) = yi (17) # {i : xi = x} i:x i =x By the same kind of law-of-large-numbers reasoning, we can be confident that r(x) → E [Y |X = x]. Unfortunately, this only works if X has only a finite set of values. If X is continuous, then in general the probability of our getting a sample at any particular value is zero, is the probability of getting multiple samples at exactly the same value of x. This is a basic issue with estimating any kind of function from data — the function will always be undersampled, and we need to fill in between the values we see. We also need to somehow take into account the fact that each yi is a sample from the conditional distribution of Y |X = xi , and so is not generally equal to E [Y |X = xi ]. So any kind of function estimation is going to involve interpolation, extrapolation, and smoothing. Different methods of estimating the regression function — different regres- sion methods, for short — involve different choices about how we interpolate, extrapolate and smooth. This involves our making a choice about how to ap- proximate r(x) by a limited class of functions which we know (or at least hope) we can estimate. There is no guarantee that our choice leads to a good ap- proximation in the case at hand, though it is sometimes possible to say that the approximation error will shrink as we get more and more data. This is an extremely important topic and deserves an extended discussion, coming next. 3.1 The Bias-Variance Tradeoff Suppose that the true regression function is r(x), but we use the function r to make our predictions. Let’s look at the mean squared error at X = x in a slightly different way than before, which will make it clearer what happens when we can’t use r to make predictions. We’ll begin by expanding (Y − r(x))2 , since the MSE at x is just the expectation of this. (Y − r(x))2 (18) 2 = (Y − r(x) + r(x) − r(x)) = (Y − r(x))2 + 2(Y − r(x))(r(x) − r(x)) + (r(x) − r(x))2 (19) that a cooked turkey is a featherless biped, to conclude that cooked turkeys are human beings. An econometrician stops there; an econometrician who wants to be famous writes a best-selling book about how this proves that Thanksgiving is really about cannibalism. 6
7 .We saw above (Eq. 16) that Y − r(x) = η, a random variable which has ex- pectation zero (and is uncorrelated with x). When we take the expectation of Eq. 19, nothing happens to the last term (since it doesn’t involve any random quantities); the middle term goes to zero (because E [Y − r(x)] = E [η] = 0), and the first term becomes the variance of η. This depends on x, in general, so let’s call it σx2 . We have MSE(r(x)) = σx2 + ((r(x) − r(x))2 (20) The σx2 term doesn’t depend on our prediction function, just on how hard it is, intrinsically, to predict Y at X = x. The second term, though, is the extra error we get from not knowing r. (Unsurprisingly, not knowing r cannot improve our predictions.) This is our first bias-variance decomposition: the total MSE at x is decomposed into a (squared) bias r(x) − r(x), the amount by which our predictions are systematically off, and a variance σx2 , the unpredictable, “statistical” fluctuation around even the best prediction. All of the above assumes that r is a single fixed function. In practice, of course, r is something we estimate from earlier data. But if those data are ran- dom, the exact regression function we get is random too; let’s call this random function Rn , where the subscript reminds us of the finite amount of data we used to estimate it. What we have analyzed is really MSE(Rn (x)|Rn = r), the mean squared error conditional on a particular estimated regression function. What can we say about the prediction error of the method, averaging over all the possible training data sets? MSE(Rn (x)) = E (Y − Rn (X))2 |X = x (21) = E E (Y − Rn (X))2 |X = x, Rn = r |X = x (22) = E σx2 + (r(x) − Rn (x))2 |X = x (23) = σx2 + E (r(x) − Rn (x))2 |X = x (24) = σx2 + E (r(x) − E Rn (x) + E Rn (x) − Rn (x))2 (25) = σx2 + (r(x) − E Rn (x) )2 + Var Rn (x) (26) This is our second bias-variance decomposition — I pulled the same trick as before, adding and subtract a mean inside the square. The first term is just the variance of the process; we’ve seen that before and isn’t, for the moment, of any concern. The second term is the bias in using Rn to estimate r — the approximation bias or approximation error. The third term, though, is the variance in our estimate of the regression function. Even if we have an unbiased method (r(x) = E Rn (x) ), if there is a lot of variance in our estimates, we can expect to make large errors. The approximation bias has to depend on the true regression function. For example, if E Rn (x) = 42 + 37x, the error of approximation will be zero if 7
8 .r(x) = 42 + 37x, but it will be larger and x-dependent if r(x) = 0. However, there are flexible methods of estimation which will have small approximation biases for all r in a broad range of regression functions. The catch is that, at least past a certain point, decreasing the approximation bias can only come through increasing the estimation variance. This is the bias-variance trade- off. However, nothing says that the trade-off has to be one-for-one. Sometimes we can lower the total error by introducing some bias, since it gets rid of more variance than it adds approximation error. The next section gives an example. In general, both the approximation bias and the estimation variance depend on n. A method is consistent2 when both of these go to zero as n → 0 — that is, if we recover the true regression function as we get more and more data.3 Again, consistency depends on how well the method matches the actual data- generating process, not just on the method, and again, there is a bias-variance trade-off. There can be multiple consistent methods for the same problem, and their biases and variances don’t have to go to zero at the same rates. 3.2 The Bias-Variance Trade-Off in Action Let’s take an extreme example: we could decide to approximate r(x) by a con- stant r0 . The implicit smoothing here is very strong, but sometimes appropri- ate. For instance, it’s appropriate when r(x) really is a constant! Then trying to estimate any additional structure in the regression function is just so much wasted effort. Alternately, if r(x) is nearly constant, we may still be better off approximating it as one. For instance, suppose the true r(x) = r0 + a sin (νx), where a 1 and ν 1 (Figure 3 shows an example). With limited data, we can actually get better predictions by estimating a constant regression function than one with the correct functional form. 3.3 Ordinary Least Squares Linear Regression as Smooth- ing Let’s revisit ordinary least-squares linear regression from this point of view. Let’s assume that the independent variable X is one-dimensional, and that both X and Y are centered (i.e. have mean zero) — neither of these assumptions is really necessary, but they reduce the book-keeping. We choose to approximate r(x) by α + βx, and ask for the best values a, b of those constants. These will be the ones which minimize the mean-squared 2 To be precise, consistent for r, or consistent for conditional expectations. More generally, an estimator of any property of the data, or of the whole distribution, is consistent if it converges on the truth. 3 You might worry about this claim, especially if you’ve taken more probability theory — aren’t we just saying something about average performance of the R, rather than any particular estimated regression function? But notice that if the estimation variance goes to zero, then by Chebyshev’s inequality each Rn (x) comes arbitrarily close to E Rn (x) with arbitrarily high probability. If the approximation bias goes to zero, therefore, the estimated regression functions converge in probability on the true regression function, not just in mean. 8
9 . 2.0 1.5 1.0 y 0.5 0.0 0.0 0.2 0.4 0.6 0.8 1.0 x ugly.func = function(x) {1 + 0.01*sin(100*x)} r = runif(100); y = ugly.func(r) + rnorm(length(r),0,0.5) plot(r,y,xlab="x",ylab="y"); curve(ugly.func,add=TRUE) abline(h=mean(y),col="red") sine.fit = lm(y ~ 1+ sin(100*r)) curve(sine.fit$coefficients[1]+sine.fit$coefficients[2]*sin(100*x), col="blue",add=TRUE) Figure 3: A rapidly-varying but nearly-constant regression function; Y = 1 + 0.01 sin 100x + , with ∼ N (0, 0.1). (The x values are uniformly distributed between 0 and 1.) Red: constant line at the sample mean. Blue: estimated function of the same form as the true regression function, i.e., r0 + a sin 100x. If the data set is small enough, the constant actually generalizes better — the bias of using the wrong functional form is smaller than the additional variance from the extra degrees of freedom. Here, the RMS error of the constant on new data is 0.50, while that of the estimated sine function is 0.51 — using the right function actually hurts us! 9
10 .error. 2 M SE(α, β) = E (Y − α − βX) (27) 2 = E (Y − α − βX) |X (28) 2 = E Var [Y |X] + (E [Y − α − βX|X]) (29) 2 = E [Var [Y |X]] + E (E [Y − α − βX|X]) (30) The first term doesn’t depend on α or β, so we can drop it for purposes of optimization. Taking derivatives, and then brining them inside the expectations, ∂M SE = E [2(Y − α − βX)(−1)] (31) ∂α E [Y − a − bX] = 0 (32) a = E [Y ] − bE [X] = 0 (33) using the fact that X and Y are centered; and, ∂M SE = E [2(Y − α − βX)(−X)] (34) ∂β E [XY ] − bE X 2 = 0 (35) Cov [X, Y ] b = (36) Var [X] again using the centering of X and Y . That is, the mean-squared optimal linear prediction is Cov [X, Y ] r(x) = x (37) Var [X] Now, if we try to estimate this from data, there are two approaches. One is to replace the true population values of the covariance and the variance with their sample values, respectively 1 yi xi (38) n i and 1 x2i (39) n i (again, assuming centering). The other is to minimize the residual sum of squares, 2 RSS(α, β) ≡ (yi − α − βxi ) (40) i 10
11 .You may or may not find it surprising that both approaches lead to the same answer: a = 0 (41) i yi xi b = 2 (42) i xi Provided that Var [X] > 0, this will converge with IID samples, so we have a consistent estimator.4 We are now in a position to see how the least-squares linear regression model is really a smoothing of the data. Let’s write the estimated regression function explicitly in terms of the training data points. r(x) = bx (43) i yi xi = x 2 (44) i xi xi = yi 2x (45) i j xj xi = yi 2 x (46) i nsX where s2X is the sample variance of X. In words, our prediction is a weighted average of the observed values yi of the dependent variable, where the weights are proportional to how far xi is from the center, relative to the variance, and proportional to the magnitude of x. If xi is on the same side of the center as x, it gets a positive weight, and if it’s on the opposite side it gets a negative weight. Figure 4 shows the data from Figure 1 with the least-squares regression line added. It will not escape your notice that this is very, very slightly different from the constant regression function; the coefficient on X is 0.004438. Visually, the problem is that there should be a positive slope in the left-hand half of the data, and a negative slope in the right, but the slopes are the densities are balanced so that the best single slope is zero.5 Mathematically, the problem arises from the somewhat peculiar way in which least-squares linear regression smoothes the data. As I said, the weight of a data point depends on how far it is from the center of the data, not how far it is from the point at which we are trying to predict. This works when r(x) really is a straight line, but otherwise — e.g., here — it’s a recipe for trouble. However, it does suggest that if we could somehow just tweak the way we smooth the data, we could do better than linear regression. 4 Eq. 41 may look funny, but remember that we’re assuming X and Y have been centered. Centering doesn’t change the slope of the least-squares line but does change the intercept; if we go back to the un-centered variables the intercept becomes Y − bX, where the bar denotes the sample mean. 5 The standard test of whether this coefficient is zero is about as far from rejecting the null hypothesis as you will ever see, p = 0.965. You should remember this the next time you look at regression output. 11
12 . 1.0 0.8 0.6 y 0.4 0.2 0.0 0.0 0.2 0.4 0.6 0.8 1.0 x abline(h=mean(all.y),lty=2) fit.all = lm(all.y~all.x) abline(a=fit.all$coefficients[1],b=fit.all$coefficients[2],col="blue") Figure 4: Data from Figure 1, with a horizontal line at the mean (dotted) and the ordinary least squares regression line (solid). If you zoom in online you will see that there really are two lines there. 12
13 .4 Linear Smoothers The sample mean and the linear regression line are both special cases of linear smoothers, which are estimates of the regression function with the following form: r(x) = yi w(xi , x) (47) i The sample mean is the special case where w(xi , x) = 1/n, regardless of what xi and x are. Ordinary linear regression is the special case where w(xi , x) = (xi /ns2X )x. Both of these, as remarked, ignore how far xi is from x. 4.1 k-Nearest-Neighbor Regression At the other extreme, we could do nearest-neighbor regression: 1 xi nearest neighbor of x w(xi , x) = (48) 0 otherwise This is very sensitive to the distance between xi and x. If r(x) does not change too rapidly, and X is pretty thoroughly sampled, then the nearest neighbor of x among the xi is probably close to x, so that r(xi ) is probably close to r(x). However, yi = r(xi )+noise, so nearest-neighbor regression will include the noise into its prediction. We might instead do k-nearest neighbor regression, 1/k xi one of the k nearest neighbors of x w(xi , x) = (49) 0 otherwise Again, with enough samples all the k nearest neighbors of x are probably close to x, so their regression functions there are going to be close to the regression function at x. But because we average their values of yi , the noise terms should tend to cancel each other out. As we increase k, we get smoother functions — in the limit k = n and we just get back the constant. Figure 5 illustrates this for our running example data.6 To use k-nearest-neighbors regression, we need to pick k somehow. This means we need to decide how much smoothing to do, and this is not trivial. We will return to this point. Because k-nearest-neighbors averages over only a fixed number of neighbors, each of which is a noisy sample, it always has some noise in its prediction, and is generally not consistent. This may not matter very much with moderately- large data (especially once we have a good way of picking k). However, it is sometimes useful to let k systematically grow √ with n, but not too fast, so as to avoid just doing a global average; say k ∝ n. Such schemes can be consistent. 6 The code uses the k-nearest neighbor function provided by the package knnflex (available from CRAN). This requires one to pre-compute a matrix of the distances between all the points of interest, i.e., training data and testing data (using knn.dist); the knn.predict function then needs to be told which rows of that matrix come from training data and which from testing data. See help(knnflex.predict) for more, including examples. 13
14 . 1.0 0.8 0.6 y 0.4 0.2 0.0 0.0 0.2 0.4 0.6 0.8 1.0 x library(knnflex) all.dist = knn.dist(c(all.x,seq(from=0,to=1,length.out=100))) all.nn1.predict = knn.predict(1:110,111:210,all.y,all.dist,k=1) abline(h=mean(all.y),lty=2) lines(seq(from=0,to=1,length.out=100),all.nn1.predict,col="blue") all.nn3.predict = knn.predict(1:110,111:210,all.y,all.dist,k=3) lines(seq(from=0,to=1,length.out=100),all.nn3.predict,col="red") all.nn5.predict = knn.predict(1:110,111:210,all.y,all.dist,k=5) lines(seq(from=0,to=1,length.out=100),all.nn5.predict,col="green") all.nn20.predict = knn.predict(1:110,111:210,all.y,all.dist,k=20) lines(seq(from=0,to=1,length.out=100),all.nn20.predict,col="purple") Figure 5: Data points from Figure 1 with horizontal dashed line at the mean and the k-nearest-neighbor regression curves for k = 1 (blue), k = 3 (red), k = 5 (green) and k = 20 (purple). Note how increasing k smoothes out the regression line, and pulls it back towards the mean. (k = 100 would give us back the dashed horizontal line.) 14
15 .4.2 Kernel Smoothers Changing k in a k-nearest-neighbors regression lets us change how much smooth- ing we’re doing on our data, but it’s a bit awkward to express this in terms of a number of data points. It feels like it would be more natural to talk about a range in the independent variable over which we smooth or average. Another problem with k-NN regression is that each testing point is predicted using in- formation from only a few of the training data points, unlike linear regression or the sample mean, which always uses all the training data. If we could somehow use all the training data, but in a location-sensitive way, that would be nice. There are several ways to do this, as we’ll see, but a particularly useful one is to use a kernel smoother, a.k.a. kernel regression or Nadaraya-Watson regression. To begin with, we need to pick a kernel function7 K(xi , x) which satisfies the following properties: 1. K(xi , x) ≥ 0 2. K(xi , x) depends only on the distance xi −x, not the individual arguments 3. xK(0, x)dx = 0 4. 0 < x2 K(0, x)dx < ∞ These conditions together (especially the last one) imply that K(xi , x) → 0 as |xi − x| → ∞. Two examples of such functions are the density of √ the Unif(−h/2, h/2) distribution, and the density of the standard Gaussian N (0, h) distribution. Here h can be any positive number, and is called the bandwidth. The Nadaraya-Watson estimate of the regression function is K(xi , x) r(x) = yi (50) i j K(xj , x) i.e., in terms of Eq. 47, K(xi , x) w(xi , x) = (51) j K(xj , x) (Notice that here, as in k-NN regression, the sum of the weights is always 1. Why?)8 What does this achieve? Well, K(xi , x) is large if xi is close to x, so this will place a lot of weight on the training data points close to the point where we are trying to predict. More distant training points will have smaller weights, 7 There are many other mathematical objects which are also called “kernels”. Some of these meanings are related, but not all of them. (Cf. “normal”.) 8 What do we do if K(x , x) is zero for some x ? Nothing; they just get zero weight in i i the average. What do we do if all the K(xi , x) are zero? Different people adopt different conventions; popular ones are to return the global, unweighted mean of the yi , to do some sort of interpolation from regions where the weights are defined, and to throw up our hands and refuse to make any predictions (computationally, return NA). 15
16 .falling off towards zero. If we try to predict at a point x which is very far from any of the training data points, the value of K(xi , x) will be small for all xi , but it will typically be much, much smaller for all the xi which are not the nearest neighbor of x, so w(xi , x) ≈ 1 for the nearest neighbor and ≈ 0 for all the others.9 That is, far from the training data, our predictions will tend towards nearest neighbors, rather than going off to ±∞, as linear regression’s predictions do. Whether this is good or bad of course depends on the true r(x) — and how often we have to predict what will happen very far from the training data. Figure 6 shows our running example data, together with kernel regression estimates formed by combining the uniform-density, or box, and Gaussian ker- nels with different bandwidths. The box kernel simply takes a region of width h around the point x and averages the training data points it finds there. The Gaussian kernel gives reasonably large weights to points within h of x, smaller ones to points within 2h, tiny ones to points within 3h, and so on, shrinking 2 like e−(x−xi ) /2h . As promised, the bandwidth h controls the degree of smooth- ing. As h → ∞, we revert to taking the global mean. As h → 0, we tend to get spikier functions — with the Gaussian kernel at least it tends towards the nearest-neighbor regression. If we want to use kernel regression, we need to choose both which kernel to use, and the bandwidth to use with it. Experience, like Figure 6, suggests that the bandwidth usually matters a lot more than the kernel. This puts us back to roughly where we were with k-NN regression, needing to control the degree of smoothing, without knowing how smooth r(x) really is. Similarly again, with a fixed bandwidth h, kernel regression is generally not consistent. However, if h → 0 as n → ∞, but doesn’t shrink too fast, then we can get consistency. Next time, we’ll look more at linear regression and some extensions, and then come back to nearest-neighbor and kernel regression, and say something about how to handle things like the blob of data points around (0.9, 0.9) in the scatter-plot. 2 2 9 Take a Gaussian kernel in one dimension, for instance, so K(xi , x) ∝ e−(xi −x) /2h . Say 2 2 xi is the nearest neighbor, and |xi − x| = L, with L h. So K(xi , x) ∝ e−L /2h , a small 2 2 −(x −x )L/2h2 −(x −x )2 /2h2 number. But now for any other xj , K(xi , x) ∝ e−L /2h e j i e j i 2 2 e−L /2h . — This assumes that we’re using a kernel like the Gaussian, which never quite goes to zero, unlike the box kernel. 16
17 . 1.0 0.8 0.6 y 0.4 0.2 0.0 0.0 0.2 0.4 0.6 0.8 1.0 x plot(all.x,all.y,xlab="x",ylab="y") axis(1,at=all.x,labels=FALSE) axis(2,at=all.y,labels=FALSE) lines(ksmooth(all.x, all.y, "normal", bandwidth=2),col="blue",lty=2) lines(ksmooth(all.x, all.y, "normal", bandwidth=1),col="red",lty=2) lines(ksmooth(all.x, all.y, "normal", bandwidth=0.1),col="green",lty=2) lines(ksmooth(all.x, all.y, "box", bandwidth=2),col="blue") lines(ksmooth(all.x, all.y, "box", bandwidth=1),col="red") lines(ksmooth(all.x, all.y, "box", bandwidth=0.1),col="green") Figure 6: Data from Figure 1 together with kernel regression lines. Solid colored lines are box-kernel estimates, dashed colored lines Gaussian-kernel estimates. Blue, h = 2; red, h = 1; green, h = 0.5; purple, h = 0.1 (per the definition of bandwidth in the ksmooth function). Note the abrupt jump around x = 0.75 in the box-kernel/h = 0.1 (solid purple) line — with a small bandwidth the box kernel is unable to interpolate smoothly across the break in the training data, while the Gaussian kernel can. 17
18 .Exercises These are for you to think through, not to hand in. 1. Suppose we use the mean absolute error instead of the mean squared error: MAE(a) = E [|Y − a|] (52) Is this also minimized by taking a = E [Y ]? If not, what value r˜ minimizes the MAE? Should we use MSE or MAE to measure error? 2. Derive Eqs. 41 and 42 by minimizing Eq. 40. 3. What does it mean for Gaussian kernel regression to approach nearest- neighbor regression as h → 0? Why does it do so? Is this true for all kinds of kernel regression? 18
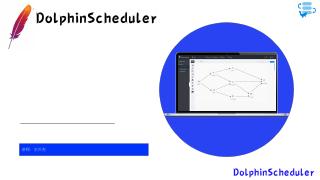
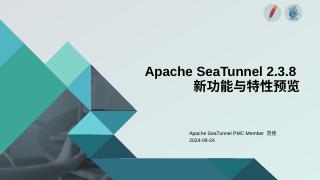
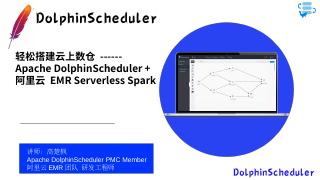
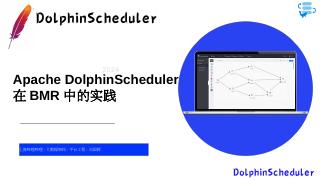
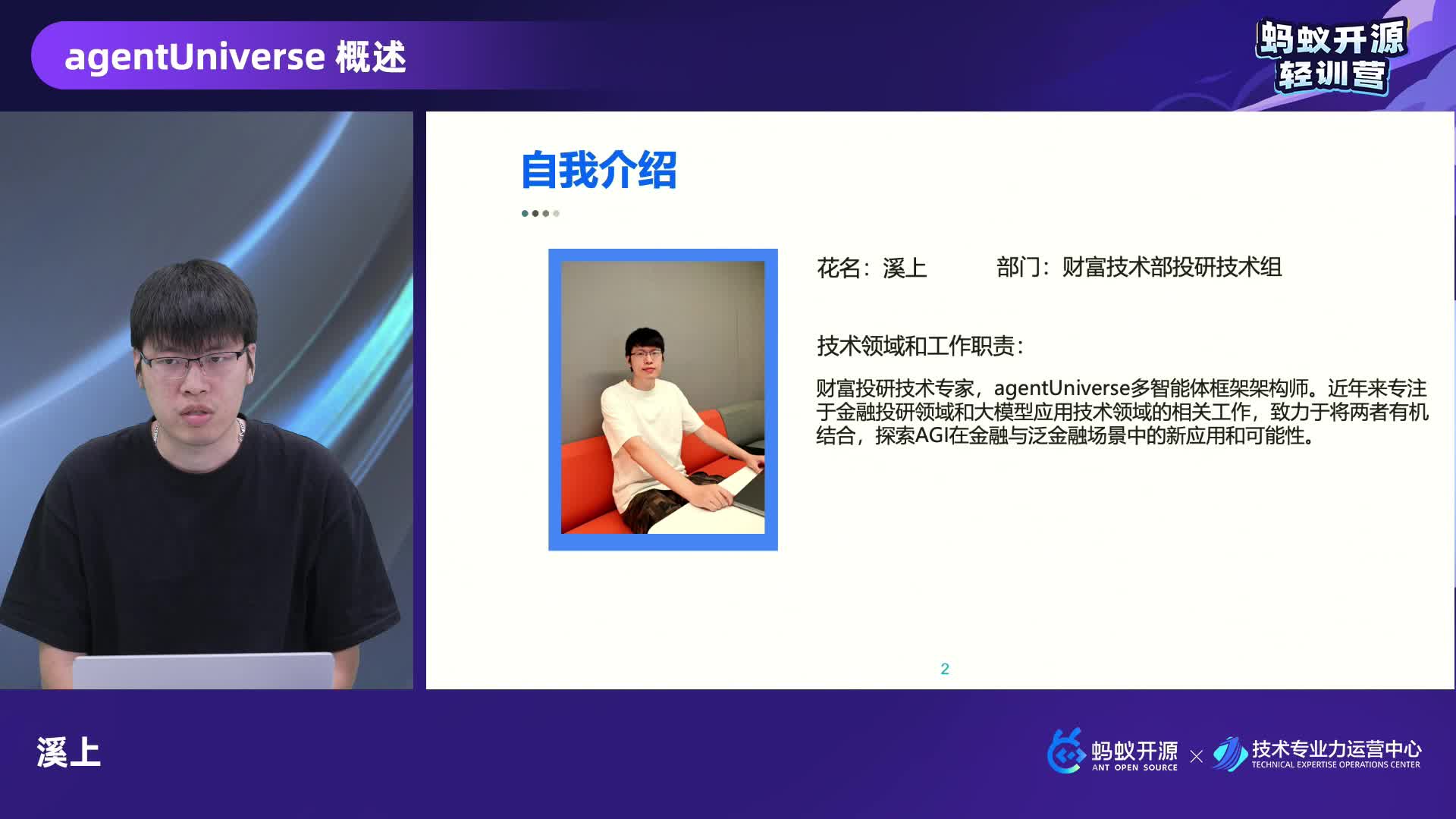
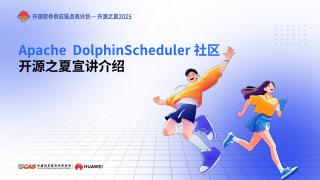
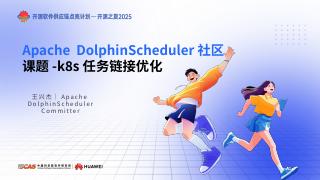
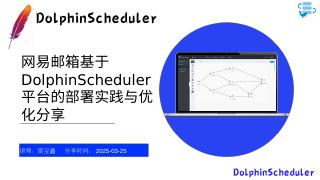