- 快召唤伙伴们来围观吧
- 微博 QQ QQ空间 贴吧
- 文档嵌入链接
- <iframe src="https://www.slidestalk.com/mlsql/MLSQLApowerfulDSLforBIgDataandAI11606?embed" frame border="0" width="640" height="360" scrolling="no" allowfullscreen="true">复制
- 微信扫一扫分享
MLSQL,A powerful DSL for BIgData and AI
展开查看详情
1 .MLSQL OverView
2 . ⾃自我简介 祝海海林林,丁⾹香园⼤大数据资深架构师 技术博客: http://www.jianshu.com/u/59d5607f1400 开源项⽬目: https://github.com/allwefantasy
3 .What’s the most successful fields for AI? Self-Driving Security and Protection
4 .And game… AlphaGo
5 .How should AI be applied ? No atom bomb Algorithm Bunch of Algorithm Empower Every Step Everyone can use
6 .00 What’s MLSQL
7 .Why design this? 1. BigData and AI should not be separated 2. A language everyone can take control 3. AI should be a easy thing
8 .Advantage Easy: SQL Script everyone can use Flexible: Code with Python/Scala Full scenes: Batch/Stream/ if you want to enhance SQL Crawler/AI
9 .Disadvantage Documents is not enough Lack of real case Actively development, API is not stable
10 .Talk Talk 01 Let’s rock DL Cifar10 机器器学习平台MLSQL实践 02 Stream My Data 03 Crawler the web 04 How we make ML real simple 05 Show me case Please Keep Quiet
11 .01 Let’s rock DL Cifar10
12 .Load and convert Images run emptyData as ImageLoaderExt.`/Users/allwefantasy/Downloads/cifar/train` where and code=''' def apply(params:Map[String,String]) = { Resize(28, 28) -> MatToTensor() -> ImageFrameToSample() } ''' as data;
13 .Extract Label from path -- convert image path to number label select split(split(imageName,"_")[1],"\\.")[0] as labelStr,features from data as newdata; train newdata as StringIndex.`${labelMappingPath}` where inputCol="labelStr" and outputCol="labelIndex" as newdata1; predict newdata as StringIndex.`${labelMappingPath}` as newdata2; select (cast(labelIndex as int) + 1) as label,features from newdata2 as newdata3;
14 .Extract Label from path(The other way) -- convert image path to number label register ScriptUDF.`` as extract_label options and lang="scala" and code=''' def apply(path:String) = { path.split(“_”)[1].split(“\\.”).first } ''' ; select extract_label(label) as labelStr,features from data as newdata; train newdata as StringIndex.`${labelMappingPath}` where inputCol="labelStr" and outputCol="labelIndex" as newdata1;
15 .Cast label to float select array(cast(label as float)) as label,features from newdata3 as newdata4;
16 .Train --train with LeNet5 model train newdata4 as BigDLClassifyExt.`${modelPath}` where fitParam.0.featureSize="[3,28,28]" and fitParam.0.classNum="10" and fitParam.0.maxEpoch="50" and fitParam.0.code=''' def apply(params:Map[String,String])={ val model = Sequential() model.add(Reshape(Array(3, 28, 28), inputShape = Shape(28, 28, 3))) 。。。。。 model.add(Dense(params("classNum").toInt, activation = "softmax").setName("fc2")) } ''';
17 .Batch Predict -- batch predict predict newdata4 as BigDLClassifyExt.`${modelPath}` as predictdata;
18 .API Predict -- deploy with api server register BigDLClassifyExt.`/tmp/bigdl` as mnistPredict; select vec_argmax(mnistPredict(vec_dense(features))) as predict_label, label from data as output;
19 .That’s all No Deploy, No Environment, People knows SQL can No Complication, do Deep Learning Now No code if you want. All is SQL-Like.
20 .02 Stream My Data
21 .Set Stream Name -- the stream name, should be uniq. set streamName="streamExample";
22 .Load no-end table from Kafka -- if you are using kafka 1.0 load kafka.`pi-content-realtime-db` options `kafka.bootstrap.servers`="---" as kafka_post_parquet; -- if you are using kafka 0.8.0/0.9.0 load kafka9.`pi-content-realtime-db` options `kafka.bootstrap.servers`="---" as kafka_post_parquet;
23 .Load no-end table from Kafka -- if you are using kafka 1.0 load kafka.`pi-content-realtime-db` options `kafka.bootstrap.servers`="---" as kafka_post_parquet; -- if you are using kafka 0.8.0/0.9.0 load kafka9.`pi-content-realtime-db` options `kafka.bootstrap.servers`="---" as kafka_post_parquet;
24 .Or We mock some Data -- mock some data. set data=''' {"key":"yes","value":"a,b,c","topic":"test","partition":0,"offset":0,"timestamp":"2008-01-24 18:01:01.001","timestampType":0} {"key":"yes","value":"d,f,e","topic":"test","partition":0,"offset":1,"timestamp":"2008-01-24 18:01:01.002","timestampType":0} {"key":"yes","value":"k,d,j","topic":"test","partition":0,"offset":2,"timestamp":"2008-01-24 18:01:01.003","timestampType":0} {"key":"yes","value":"m,d,z","topic":"test","partition":0,"offset":3,"timestamp":"2008-01-24 18:01:01.003","timestampType":0} {"key":"yes","value":"o,d,d","topic":"test","partition":0,"offset":4,"timestamp":"2008-01-24 18:01:01.003","timestampType":0} {"key":"yes","value":"m,m,m","topic":"test","partition":0,"offset":5,"timestamp":"2008-01-24 18:01:01.003","timestampType":0} '''; -- load data as table load jsonStr.`data` as datasource; -- convert table as stream source load mockStream.`datasource` options stepSizeRange="0-3" and valueFormat="csv" and valueSchema="st(field(column1,string),field(column2,string),field(column3,string)) " as newkafkatable1;
25 .Processing -- aggregation select column1,column2,column3,kafkaValue from newkafkatable1 as table21;
26 .Save Stream save append table21 as newParquet.`/table1/hp_stat_date=${date.toString("yyyy-MM- dd")}` options mode="Append" and duration="30" and checkpointLocation="/tmp/ckl1";
27 . That’s all,No select ..... as table1; -- register watermark for table1 register WaterMarkInPlace.`table1` as tmp1 options eventTimeCol="ts" and delayThreshold="1 seconds"; We also support watermark -- process table1 select count(*) as num from table1 group by window(ts,"30 minutes","10 seconds") as table2; save append ......
28 .03 Crawl the web
29 .How to load link list from web page load crawlersql.`https://www.csdn.net/nav/ai` options matchXPath="//ul[@id='feedlist_id']//div[@class='title']//a/@href" and fetchType="list" and `page.type`="scroll" and `page.num`="10" and `page.flag`="feedlist_id" as aritle_url_table_source;
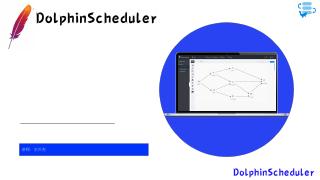
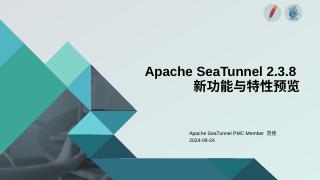
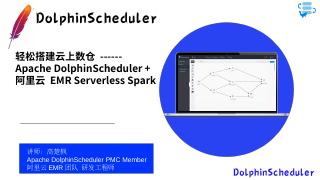
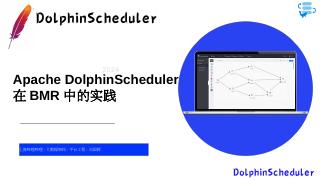
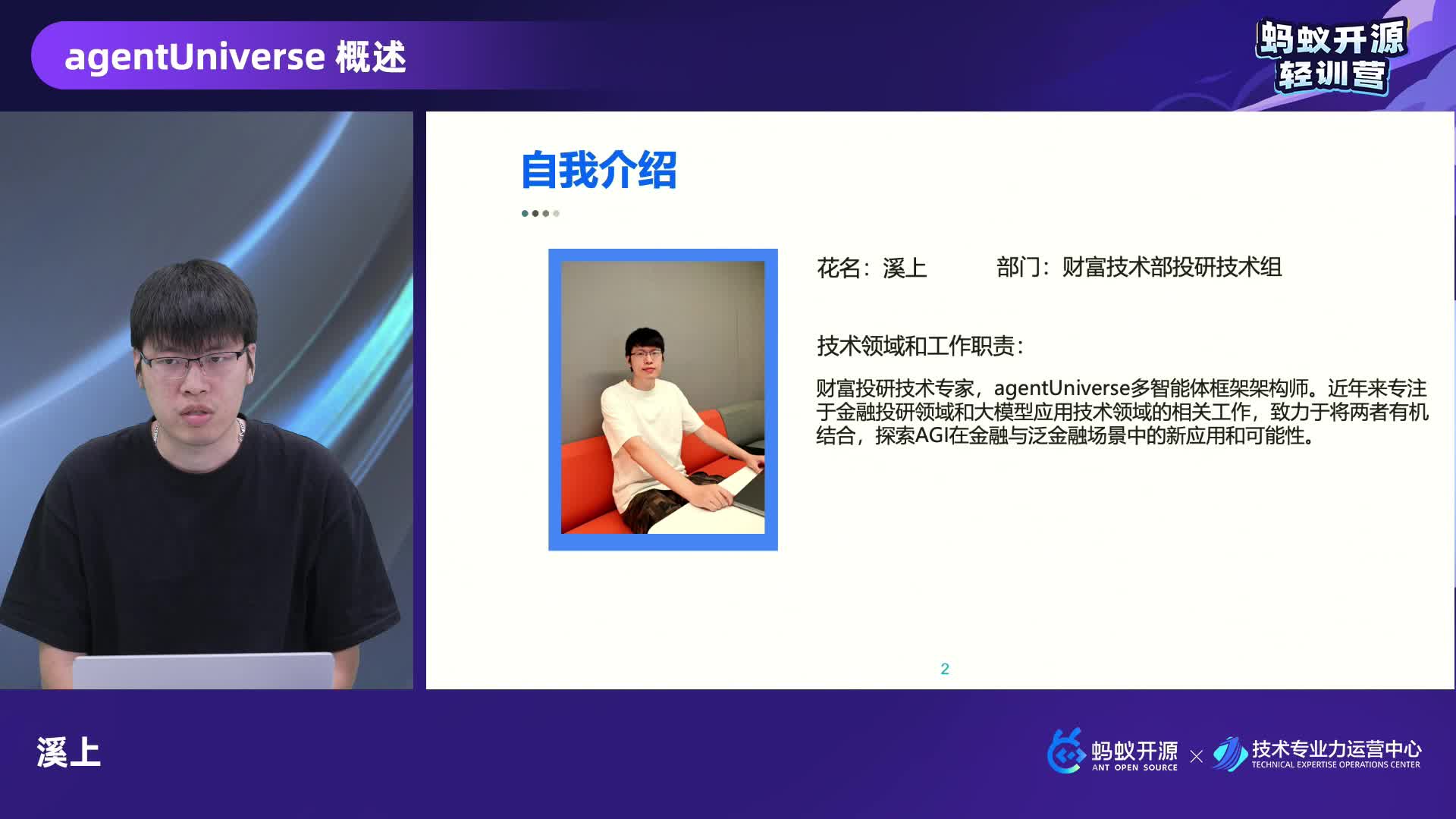
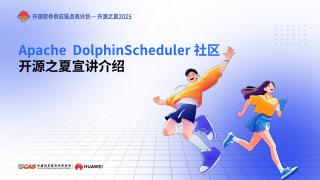
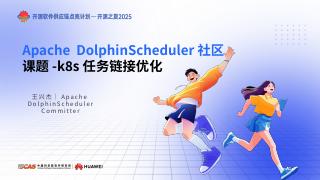
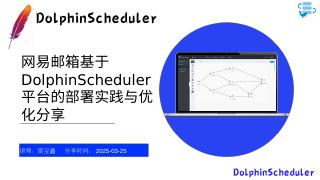