- 快召唤伙伴们来围观吧
- 微博 QQ QQ空间 贴吧
- 文档嵌入链接
- <iframe src="https://www.slidestalk.com/Spark/Real_Time_Analytics_and_Actions_Across_Large_Data_Sets_with?embed" frame border="0" width="640" height="360" scrolling="no" allowfullscreen="true">复制
- 微信扫一扫分享
Real-Time Analytics and Actions Across Large Data Sets with Apache Spark
展开查看详情
1 .Real-Time Analytics & Actions at Scale with Apache Spark and Nuclio (Serverless) Yaron Ekshtein, Iguazio April 2019
2 .Agenda § Current data-science and analytics challenges § A continuous and cloud native architecture § What does Serverless have to do with it? § Use cases § Summary and Q&A
3 . The Surprising Truth About What it Takes to Build a Machine Learning Product Source: https://medium.com/thelaunchpad/the-ml-surprise-f54706361a6c Josh Cogan, Google 3
4 .The Data-Driven Business Challenge From Reactive to Proactive and Intelligent Event-Driven Value of Data Interactive Batch Real-time Minutes Days Time to Action
5 .Evolve Into an Agile Cloud-Native Architecture Your Business Logic Innovate Any Containerized Microservice Consume Cloud Storage and Databases
6 . Today: Intelligent App Pipeline is Complex and Siloed Multiple Management Interfaces: Collection and ML Development Deployment & Serving Exploration and Training (cloud or edge) Data Sources Stream Processing Interactive Data Science Triggers and ML model Interaction Data Lakes/ ETL and Batch ML Training Jobs Interactive app Warehouses Reports and Dashboards Data and Data and Data and Compute: Compute: Compute: App Developers, Data Engineers Data Scientists Data Engineers 6
7 . A Continuous Pipeline, Focused On Production Develop Deploy Monitor Collect, Explore Train and Test Deploy with and Tag Data ML Models Serverless Triggers and Data Sources Interactions Monitor Microservices Real-time and historical data 7
8 . Nuclio: Taking Serverless to The Next Level Extreme Performance Advanced Data & AI Features Statefulness nuclio processor Shard 1 Workers Shard 2 Event Function Shard 3 Workers Listeners Workers Functions Shard 4 DB, MQ, File Workers § Zero copy, buffer reuse § Auto-rebalance, checkpoints § Data bindings § Up to 400K events/sec/proc § Any trigger source § Shared volumes § GPU Support § Simple integration § Context cache Open-source Serverless for compute & data intensive tasks 8
9 .Use-cases: Building Real-Time Intelligent Apps, The Easy way !
10 . Demo Video https://www.youtube.com/watch?v=vA8Uq7MvxL4 10
11 . Demo: Voice Driven Real-Time Analytics GOOGLE MAP SERVICE Update Voice Locations Query SMART HOME DEVICE SQL Query AI SQL API WEB UI (REACT)
12 . Use Case: Real-Time Analysis of Financial Data Data Exploration & RT Analysis Real-time Dashboard RT Tweet Sentiment Analysis Tick feed Analysis World Trading Data & Tagging News Stream • Enriched tweet stream viewer • Stocks tables • Stocks + sentiment TSDB 12
13 . Auto-Healing Network Operations Predict network outages and avoid them in real-time § Cross correlating real time data from multiple sources with historical data § AI based predictions trigger pre-programmed actions that fix evolving problems in the network § Implemented within weeks 13
14 . Demo: Predictive Netops Using Serverless + Spark Auto-deploy Exploration & ML Training, Correlation Model export NLP processing Of real-time Failure & Anomaly Serverless router logs Spark prediction NetFlow data Real-time DB Real-time telemetry 14
15 . Real-time Data and AI for Airport Operations Leading Airport Ground Operations uses AI to react faster to schedule changes § Quicker ground handling response to flight re-scheduling § Operational efficiency and visibility Flight Status Real-time Apps Ingest and Process Data for Intelligent Apps Dashboards Passenger Push / Pull via alerts and actions status REST API Vehicle Insights Telemetry BI style dashboards & alerts Staff Events Streams roster Real-time Database Intelligent Apps Baggage Other AI/ML NoSQL + K/V tables + TSDB status Systems Flight Schedule Scheduled batch 15
16 .Example: Predictive Maintenance Based on Real-time + Historical Data Real-time Predicted Devices & Machines Alerts Alerts Process Stream Predict Every 15 Upload to Every 6 Web Update ML Sensor Data Trigger minutes Cloud hours Model hook Aggregate using Time Series APIs Query APIs • ML Models NoSQL & Time • Machine Metadata Real-time Series API Time Series Vectors • Environmental data dashboard (Avg, Min/Max, Stdev per sensor) intelligent edge
17 . Summary Build continuous, AI-driven and proactive apps faster § Focus on using data, not collecting it § Adopt a continuous data and integration approach § Consolidate cloud-native microservices architecture § Use Serverless – for faster agile results My Email: yarone@Iguazio.com 17
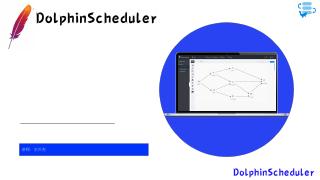
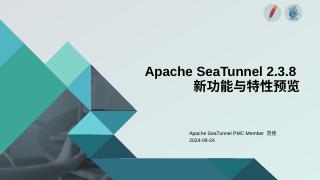
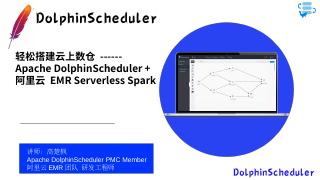
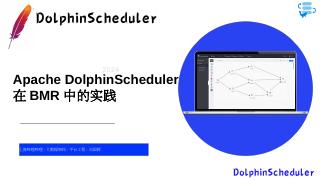
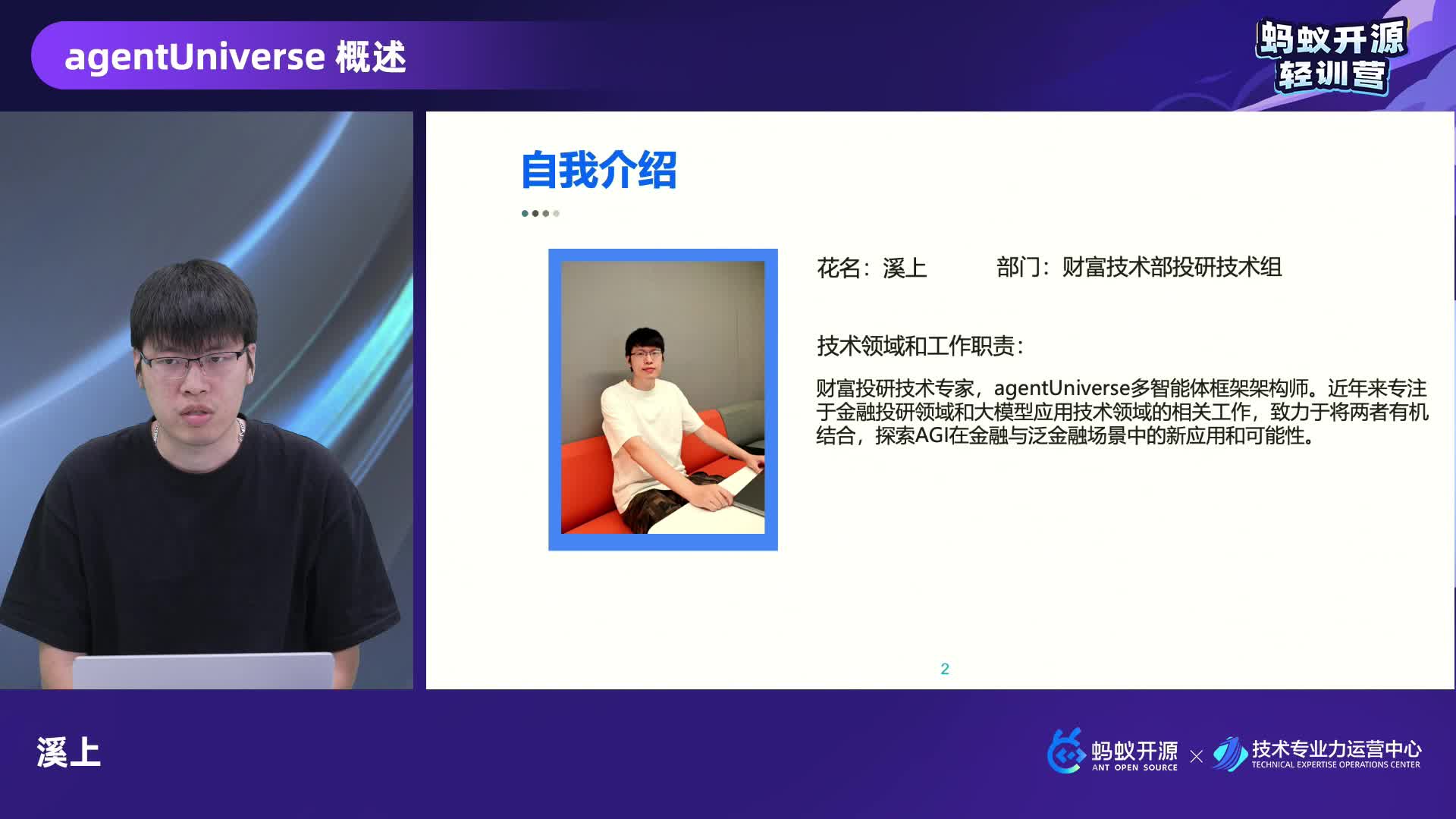
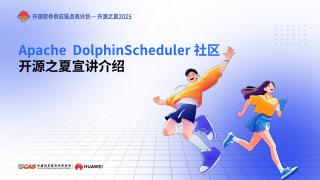
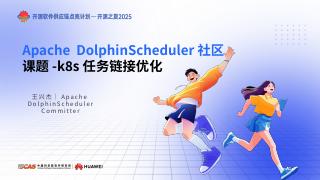
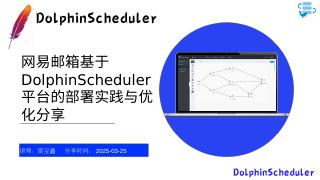